The Art of Deception: Unlocking the Secrets of Realistic Face Swaps with AI
Have you ever wanted to see your favorite celebrities in a different movie role or face swaps with a friend just for fun? AI-powered face swapping has made this possible with just a few clicks, delivering surprisingly realistic results.
But face swapping isn’t just for entertainment. It plays a huge role in film production, advertising, and even law enforcement, helping create seamless deepfakes, enhance visual effects, and assist in forensic investigations.
In this guide, we’ll explore the best AI face swap tools and techniques, breaking down how they work and how you can create high-quality, realistic swaps. Whether you’re a content creator, filmmaker, or AI enthusiast, this post will help you understand the process and the latest advancements in AI-powered face swapping.
Let’s get started!
Overview of AI Face Swaps Technology
AI face swap technology is a game-changing innovation that has transformed how we edit and manipulate faces in images and videos. It relies on machine learning and deep learning algorithms to swap faces with incredible accuracy and realism.
Key Components
- Face Detection: Identifies and locates faces within an image or video
- Facial Landmarks: Pinpoints specific facial features, such as eyes, nose, mouth, and jawline
- Convolutional Neural Networks (CNNs): Analyzes and extracts facial features, including skin texture, hair, and facial expressions
- 3D Facial Modeling: Creates a 3D model of the face, enabling manipulation in 3D space

The Face Swaps Process
- Face Extraction: Extracts the facial features from one image or video
- Face Transfer: Transfers the extracted facial features to another image or video
- Blending: Seamlessly blends the transferred face with the target image or video
This technology has far-reaching implications for various industries, from entertainment and advertising to law enforcement and security. In the next section, we’ll explore the techniques and tools used in AI face swap technology.
Revolutionizing Face Swapping with AI
Artificial intelligence has reshaped countless industries, and face swapping is no exception. By using advanced algorithms and large-scale data analysis, AI-powered face swaps have become more realistic and precise than ever. This technology analyzes vast datasets, learning to recognize facial features, expressions, angles, and lighting conditions to create seamless and natural-looking swaps.
The AI Learning Process

The journey begins with feeding the AI a vast dataset of images, allowing it to learn and identify key facial features such as:
- Eyes
- Nose
- Mouth
- Jawline
With this knowledge, the AI can then swap faces, preserving the subtleties of the original face, including:
- Subtle expressions
- Skin textures
Seamless Integration
The AI seamlessly integrates the original face onto another person’s face, creating a remarkably realistic result.
Expanding Possibilities
As AI technology advances, the applications of ai face changing are growing, including:
- Creating hyper-realistic deepfakes
- Preserving the legacy of actors
- Opening up new possibilities
Understanding AI Face Swaps
AI face swapping involves transferring one person’s face onto another’s body using advanced deep learning algorithms to create natural and convincing results. Unlike traditional methods, AI-powered tools use deep learning models trained on large datasets of facial images. These models accurately detect and replicate facial features, preserving expressions, skin tone, and lighting for a realistic appearance.
Thanks to AI-driven face swap technology, the swapped face adapts seamlessly to different angles and movements, ensuring that the final output looks smoothly integrated into the original image or video.
How AI Face Swapping Differs from Traditional Image Editing
Aspect | Traditional Image Editing | AI Face Swapping |
---|---|---|
Process | Manual cut-and-paste techniques | Uses sophisticated AI algorithms to analyze and swap faces |
Challenges | Mismatched skin tones, awkward blending, unnatural expressions | Automatically handles these issues for natural and coherent results |
Dynamic Content | Struggles with consistency in videos, requires manual effort | Maintains consistency across frames, even with movement and expression changes |
Precision & Customization | Limited by manual capabilities | Adapts to various facial structures and lighting conditions with high accuracy |
Result | Often unconvincing and visibly altered | Highly realistic, making the swap appear as part of the original scene |
How Does AI Face Swaps Work?
AI face swapping uses deep learning models, a branch of artificial intelligence that mimics how the human brain processes information. These models are trained on huge datasets containing thousands or even millions of facial images. Through this training, AI learns to identify and replicate facial features like the eyes, nose, mouth, and overall structure, ensuring a realistic swap.
Once trained, the AI takes two faces—one from the source image and one from the target image—and blends them together in a way that looks natural. But how does it actually do this? Let’s break it down step by step.
Key Steps in AI Face Swaps
Face Detection and Alignment
The first step in AI face swapping is detecting and aligning faces in both the source and target images. Face detection identifies where a face is located, while face alignment ensures both faces are positioned similarly. This step is crucial because even a small misalignment can make the swap look unnatural.
A common technique used for alignment is facial landmark detection. This method identifies key points on the face, such as the corners of the eyes, the tip of the nose, and the edges of the mouth. These landmarks help the AI understand the face’s structure, making sure the swap looks smooth and realistic.
Feature Extraction
Once the faces are aligned, the AI moves to feature extraction. This step analyzes the source face, identifying key details like the shape of the eyes, curve of the lips, and contour of the jawline. These features are then mapped onto the target face to create a natural-looking swap.
But the AI doesn’t just copy and paste these features. It also takes into account lighting, skin tone, and facial expressions to make sure everything blends seamlessly. This is where the real magic happens—by understanding how light interacts with the face and how expressions change, AI creates a swap that looks lifelike and realistic.
Facial Blending
After mapping the key facial features, the AI blends the source face with the target face. This step is crucial for making the swap look natural rather than like a simple cut-and-paste job.
Facial blending is where the AI fine-tunes skin tone, lighting, and texture to seamlessly merge the two faces. It ensures that the swap appears as if it was always part of the original image. To make the transition even smoother, the AI may apply color correction and detail enhancement, matching elements like wrinkles, shadows, and highlights so that everything looks consistent and realistic.
Post-Processing for Enhanced Realism
Finally, the AI applies post-processing techniques to fine-tune the face swap. This can include adjusting contrast, brightness, and texture to ensure the new face blends naturally with the rest of the image. In videos, the AI ensures the swap remains consistent across frames, even as the subject moves or changes expression.
This step adds the final touches that make the swap truly convincing. By carefully refining every detail—such as matching the grain of the original image or smoothing out minor imperfections—the AI ensures that the result looks seamless and realistic.
Key Techniques for Realistic Face Swaps
To achieve the most realistic face swaps, several key techniques are employed:
- Deep Learning Models: These models are trained on extensive datasets, allowing the AI to learn and replicate facial features with remarkable accuracy.
- Generative Adversarial Networks (GANs): GANs are used to generate high-quality images that closely resemble real faces. They play a crucial role in ensuring that the swapped face looks natural.
- Facial Landmark Detection: By identifying key points on the face, this technique helps the AI align the faces correctly and maintain the natural geometry of the face.
- Color Correction and Blending: Matching the skin tone and lighting between the source and target faces is essential for a convincing swap. The AI carefully blends the features to ensure a smooth transition.
- Post-Processing: Final adjustments, such as noise addition and detail enhancement, are applied to make the swap look as though it was always part of the original image or video.
By combining these techniques, AI face swapping creates results that are not only realistic but also adaptable to various situations. Whether it’s a still image or a moving video, AI face swapping can handle it all with ease.
Must Read
- Escape Sequences and Raw Strings in Python: The Complete Guide
- Prime Factorization Algorithm in Python
- How to Get the First Digit of a Number in Python
- Global and Local Variables in Python: A Complete Guide
- How to Return Multiple Values from a Function in Python
Tools and Libraries for AI Face Swaps
When it comes to AI face swapping, using the right tools and libraries is important for achieving high-quality results. These tools simplify the process and offer various features to help you create impressive swaps. One of the most popular and powerful options in this space is DeepFaceLab.
Let’s explore why DeepFaceLab is a top choice for face swapping and how you can get started with it.
DeepFaceLab
DeepFaceLab is a powerful tool that’s widely used for creating high-quality face swaps and deepfakes. It comes with a comprehensive set of features, making it ideal for users who want to achieve detailed and realistic results. Whether you’re a beginner or an advanced user, DeepFaceLab offers various options to help you bring your creative visions to life.
Features and Use Cases
DeepFaceLab stands out for its flexibility and advanced capabilities. Here are some of its key features:
- Face Extraction and Alignment: DeepFaceLab can automatically detect and extract faces from images or videos, ensuring they are accurately aligned for better swaps. This is crucial for ensuring that the swapped faces match in position and orientation.
- Training and Fine-Tuning: The tool allows you to train models with your own datasets, giving you full control over the quality and style of the face swaps. You can fine-tune these models to handle different lighting conditions or facial expressions.
- Multiple Algorithms: DeepFaceLab supports a variety of algorithms for tasks like face swapping, facial expression transfer, and more. This gives you the flexibility to choose the best approach for your specific project.
- User-Friendly Interface: Despite offering advanced features, DeepFaceLab has a relatively user-friendly interface, making it accessible to those who may not be experts in AI but still want to experiment with face swapping.
DeepFaceLab is commonly used for a range of applications, including:
- Entertainment: Creating realistic face swaps for movies, TV shows, or online videos.
- Educational Content: Demonstrating AI capabilities in a visual and engaging manner.
- Artistic Projects: Exploring creative possibilities in digital art and media.
How to Get Started
Getting started with DeepFaceLab involves a few key steps:
- Download and Installation:
Visit the official DeepFaceLab repository on platforms like GitHub to download the latest version. The installation process may require setting up dependencies and configuring your environment, so be sure to follow the detailed instructions provided in the repository carefully. - Prepare Your Data:
Before starting, gather the images or videos you want to use. Ensure the sources are of high quality and properly aligned to get the best results. - Face Extraction and Alignment:
Use DeepFaceLab’s tools to extract and align faces from your data. This ensures that the faces are correctly positioned and oriented for the swapping process. - Train Your Model:
Depending on your project, you may need to train a model using your data. This step involves configuring training parameters and allowing DeepFaceLab to process your data, creating a model capable of performing face swaps. - Perform the Swap:
With your trained model, proceed with the face swapping process. DeepFaceLab offers various options to customize the output, so you can adjust settings and achieve the desired results. - Post-Processing:
After the face swap, you may need to do some post-processing to refine the result. This could involve adjusting colors, blending edges, and ensuring the swapped face integrates smoothly with the original image.
By following these steps, you’ll be ready to experiment with DeepFaceLab and create realistic and engaging face swaps.
FaceSwap
If you’re diving into AI face swapping, FaceSwap is another excellent tool you should consider. Known for its user-friendly approach and powerful features, FaceSwap makes it easier to achieve high-quality face swaps. Let’s take a closer look at what makes FaceSwap a standout choice and how you can get started with it.
Overview and Installation
FaceSwap is a powerful, open-source tool that makes face swapping simple and easy. It lets users create realistic face swaps and deepfakes using advanced AI technology. Whether you’re a beginner or an experienced user, FaceSwap is designed to be easy to use, with lots of features that don’t make things too complicated. It’s a great option for anyone who wants to try face swapping without feeling overwhelmed.
Installing FaceSwap involves a few simple steps:
- Download FaceSwap:
Start by downloading the tool from the official FaceSwap website or its repository, often available on GitHub. Be sure to get the latest version to take advantage of the newest updates and improvements. - Check System Requirements:
Make sure your computer meets the necessary specifications. FaceSwap typically requires a powerful GPU for the best performance, along with enough RAM and storage. - Setup and Configuration:
Follow the installation instructions provided by FaceSwap. You may need to set up Python and install some libraries, as well as configure environment settings. The installation guide will help you step by step, showing how to install dependencies and configure everything correctly. - Prepare Your Data:
Before starting your face swap project, gather your images or videos. Make sure they are high-quality and aligned properly to get the best results. FaceSwap provides tools to help with face extraction and alignment, ensuring your data is ready for the swap.
Key Features and Applications of FaceSwap
FaceSwap comes with a variety of features designed to make face swapping simple and effective. Here are some of its key features:
- Intuitive Interface:
FaceSwap offers an easy-to-use interface that makes the face-swapping process simple. Even if you’re not an expert, the tool’s design helps you navigate through tasks with ease. - Face Extraction and Alignment:
The tool includes features for extracting and aligning faces from images or videos. Getting accurate face alignment is crucial for a realistic swap, and FaceSwap ensures this step is easy to carry out. - Training Models:
FaceSwap lets you train your own models using your data. This feature gives you the flexibility to customize the model based on your specific needs, making sure the face swaps turn out just the way you want. - Variety of Algorithms:
The tool supports different algorithms for face swapping and related tasks. This allows you to pick the best method for your project, whether you’re working with images or videos. - Community Support:
As an open-source project, FaceSwap is supported by an active community of users and developers. You can find a lot of resources, like tutorials, forums, and troubleshooting advice, making it easier to learn and solve any issues you may encounter.
Open Source Libraries and Frameworks
When working on AI face swapping projects, using open-source libraries can make your job easier. These tools offer a variety of features, from detecting faces to creating custom models for swapping. Let’s take a look at three important libraries—OpenCV, dlib, and TensorFlow/PyTorch—and see how they can help in face swapping tasks.
- OpenCV:
OpenCV is a popular tool for computer vision tasks, like detecting and processing faces. It’s great for working with images and videos in real-time. You can use OpenCV to detect faces, align them properly, and adjust the size of the images to fit your model. It’s simple to use and helps you get things done quickly. - dlib:
dlib is another helpful tool that specializes in machine learning and computer vision. It’s especially good at detecting facial landmarks—key points on the face, such as the eyes, nose, and mouth. This helps make sure the faces are properly aligned before swapping them. dlib has pre-trained models that can automatically detect faces, making it easier for you to work with face data. - TensorFlow/PyTorch:
TensorFlow and PyTorch are both deep learning tools used to build and train AI models. You can use them to create custom models that learn how to swap faces. These libraries allow you to train your models to be very accurate and can speed up the process with GPU support. TensorFlow and PyTorch give you the flexibility to create models that suit your specific face swapping needs.
Using these libraries, you can make the face swapping process easier and more effective. Whether you’re detecting faces, aligning them, or training models, these tools help you create high-quality results for your projects.mentation and innovation, enabling you to push the boundaries of what’s possible in face swaps technology.
Step-by-Step Guide to Implementing AI Face Swaps
Creating an AI face swaps project involves several key steps, from setting up your environment to preparing your dataset. Here’s a detailed guide to help you get started and ensure a smooth workflow for your face swapping project.
Setting Up Your Environment
To get started with AI face swapping, you need to install the important tools and libraries:
- Python: Ensure Python is installed on your system as it’s the primary language for AI projects. Download it from the official Python website.
- TensorFlow: Install TensorFlow for building and training machine learning models by running:
pip install tensorflow
3. OpenCV: Use OpenCV for image and video processing with:
pip install opencv-python
4. dlib: For face landmark detection and alignment, install dlib using:
pip install dlib
Ensure all libraries are compatible with your operating system and Python version. Check the documentation if you face any issues.
Importing Libraries
Before you begin writing your face swap code, you’ll need to import the necessary libraries. These libraries provide the tools you’ll use for image processing, face detection, and machine learning.
Here’s how you can import the essential libraries:
import cv2
import dlib
import numpy as np
from tensorflow.keras.models import load_model
- cv2 (OpenCV): Handles image processing tasks such as loading images, face detection, and applying transformations.
- dlib: Detects facial landmarks (key points on the face) for accurate alignment and fitting of faces.
- numpy: Performs numerical operations and manages array data for image manipulation.
- tensorflow.keras.models: Loads and uses pre-trained machine learning models to generate or modify faces.
These libraries collectively provide the tools needed for image processing, face detection, and model-based transformations in face swapping projects.
Once you have the libraries imported, you’ll need to proceed with the following steps:
Loading and Preparing Images
Getting your images ready is an important first step in any face swapping project. This ensures your images are in the right format and ready for tasks like detecting faces and editing them. Let’s look at how you can load and prepare images using OpenCV in a simple way.
Python Code for Loading and Preparing Images
Here’s a simple function to load an image from a file path and prepare it for further processing:
def load_image(image_path):
# Load the image from the specified path
image = cv2.imread(image_path)
# Convert the image from BGR (OpenCV format) to RGB (common format)
image = cv2.cvtColor(image, cv2.COLOR_BGR2RGB)
return image
- Loading the Image:
image = cv2.imread(image_path)
Reads the image from the specified file path in BGR format (OpenCV default).
2. Converting Color Format:
image = cv2.cvtColor(image, cv2.COLOR_BGR2RGB)
Converts the image from BGR to RGB format for compatibility with other libraries and accurate color representation.
3. Returning the Image:
return image
Returns the image in RGB format, ready for further processing.
By following these steps, you ensure the image is correctly formatted and prepared for face detection and transformations in your face swapping project.
Why This Matters
Preparing your images correctly is the first step to making sure your face swap looks natural. By converting the images to RGB format, you ensure they work properly with the tools and models you’ll use later. This simple step is essential for the entire face-swapping process.
Once your images are ready, you can move on to detecting faces, identifying key points, and making the necessary adjustments before performing the face swap.
Face Detection and Alignment
Python Code for Face Detection
The following code demonstrates how to set up face detection and align facial features:
import dlib
import cv2
# Load the pre-trained face detector and shape predictor
detector = dlib.get_frontal_face_detector()
predictor = dlib.shape_predictor('shape_predictor_68_face_landmarks.dat')
def detect_face(image):
# Convert the image to grayscale as face detection works better with single channel images
gray = cv2.cvtColor(image, cv2.COLOR_RGB2GRAY)
# Detect faces in the grayscale image
faces = detector(gray)
return faces
Detailed Explanation
1. Loading the Face Detector and Shape Predictor:
detector = dlib.get_frontal_face_detector()
predictor = dlib.shape_predictor('shape_predictor_68_face_landmarks.dat')
dlib.get_frontal_face_detector()
initializes a pre-trained face detector that can identify faces in images.dlib.shape_predictor('shape_predictor_68_face_landmarks.dat')
loads a pre-trained model for detecting facial landmarks.- Using these pre-trained models allows you to quickly and accurately detect faces and facial landmarks without needing to train your own models.
2. Converting Image to Grayscale:
gray = cv2.cvtColor(image, cv2.COLOR_RGB2GRAY)
- This line converts the image from RGB to grayscale. Face detection algorithms often work more effectively with grayscale images because they simplify the image data, focusing only on the intensity of light rather than color.
- Grayscale images reduce computational complexity and enhance the accuracy of face detection by providing a single channel image where face features can be more easily identified.
3. Detecting Faces:
faces = detector(gray)
- The
detector
function identifies faces in the grayscale image. It returns a list of rectangles where faces are detected. - Detecting faces accurately is the first step in any face swapping project. These rectangles define the areas of the image that contain faces and will be used for further processing.
4. Returning Detected Faces:
return faces
- This line returns the list of detected faces. Each face is represented by a rectangle that encloses the face in the image.
- Returning these face regions allows you to proceed with tasks like aligning faces, detecting landmarks, and ultimately performing the face swap.
Face Swapping Logic
Face swapping involves more than just placing one face onto another. It requires careful manipulation to ensure the faces blend naturally and look realistic. Here’s a detailed breakdown of how you might implement face swapping in your code.
Python Code for Face Swapping
Here’s an example function for face swapping, with an outline of the steps involved:
def swap_faces(image1, image2):
# Example function for swapping faces
# Detailed implementation required here
pass
Detailed Explanation
- Overview of Face Swapping:Face swapping involves several steps:
- Detect and Extract Faces: Identify faces in both images and extract the regions containing the faces.
- Align Faces: Ensure that the faces are aligned properly by matching facial landmarks.
- Swap Faces: Replace the face from one image with the face from the other image.
- Blend Faces: Make the swapped face blend naturally with the original image by adjusting colors, lighting, and edges.
- Detect and Extract Faces:
- Detect Faces: Use the face detection function to find and extract the face regions from both images.
- Extract Face Regions: Crop the detected face regions from the images. This step involves isolating the area of the face to be swapped.
def extract_face(image, face_rect):
x, y, w, h = (face_rect.left(), face_rect.top(), face_rect.width(), face_rect.height())
face = image[y:y+h, x:x+w]
return face
3. Align Faces:
- Detect Facial Landmarks: Use a shape predictor to identify key facial features like the eyes, nose, and mouth.
- Align Faces: Transform the face to match the alignment of the target face. This might involve resizing, rotating, and warping the face to fit the target image.
def align_face(face, landmarks):
# Example function to align face based on landmarks
# Detailed implementation required here
pass
4. Swap Faces:
- Place Face in Target Image: Position the extracted and aligned face onto the target image. Ensure that the face fits well within the target’s facial region.
- Adjust Position and Size: Resize and adjust the face to match the target face’s dimensions and position.
def place_face(target_image, face, position):
x, y = position
# Resize face to match the target face size
# Paste face onto target image at the specified position
target_image[y:y+face.shape[0], x:x+face.shape[1]] = face
return target_image
5. Blend Faces:
- Blend Edges: Use techniques like feathering to blend the edges of the swapped face with the target image. This helps make the swap less noticeable.
- Adjust Color and Lighting: Match the color and lighting of the swapped face with the target image to ensure consistency and realism.
def blend_faces(target_image, face, position):
# Example function to blend face with target image
# Detailed implementation required here
pass
Post-Processing and Saving the Result
After finishing the face swap, the last step is to save the result properly and apply any needed post-processing. This ensures the final image looks its best and is ready to be used or shared. Let’s walk through how to handle this step in detail.
Python Code for Saving the Result
Below is a function for saving the final image after the face swap is complete:
def save_image(image, output_path):
# Convert the image from RGB to BGR
image_bgr = cv2.cvtColor(image, cv2.COLOR_RGB2BGR)
# Save the image to the specified path
cv2.imwrite(output_path, image_bgr)
Detailed Explanation
- Converting the Image Format:
image_bgr = cv2.cvtColor(image, cv2.COLOR_RGB2BGR)
- The
cvtColor
function converts the image from RGB (Red, Green, Blue) to BGR (Blue, Green, Red) color format. This is important because OpenCV’simwrite
function, which saves the image to a file, expects images in BGR format. - Color conversion ensures that the colors in your final image are displayed correctly when viewed or shared. If you skip this step, the colors in your saved image might appear incorrect or distorted.
2. Saving the Image:
cv2.imwrite(output_path, image_bgr)
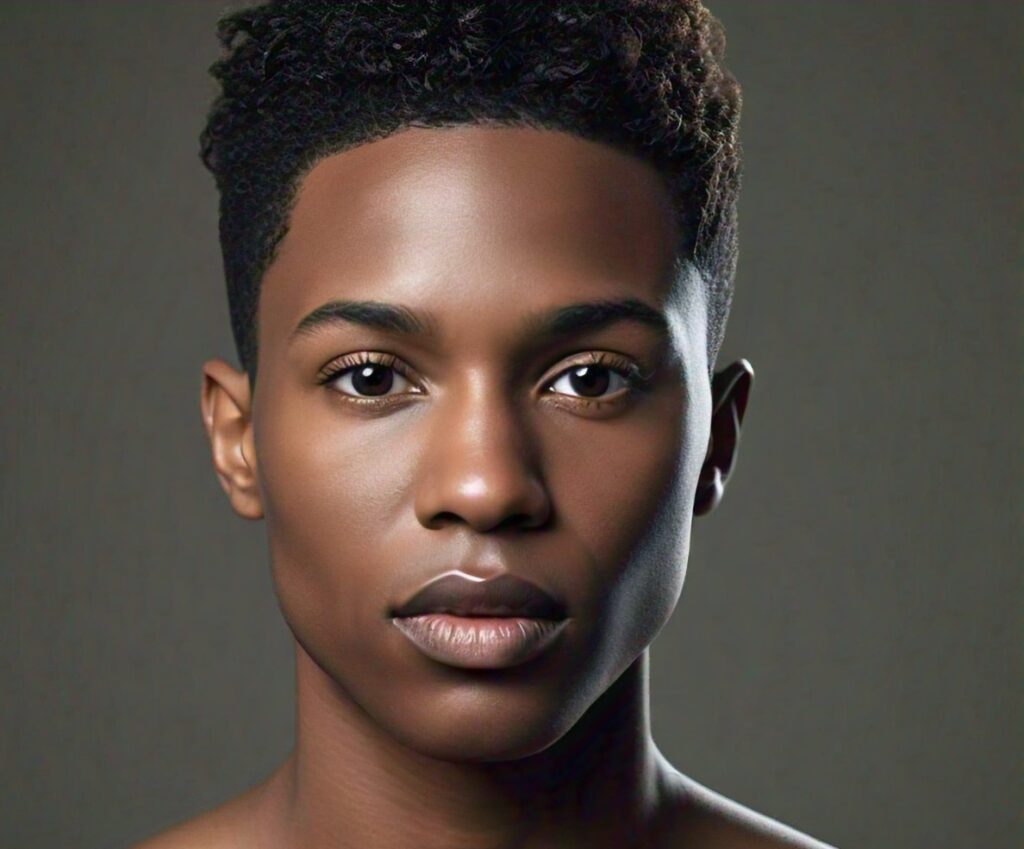
- The
imwrite
function saves the image to the specified file path (output_path
). It writes the image data to the file in the format specified by the file extension (e.g., JPEG, PNG). - Saving the image allows you to preserve the final result of your face swapping project. This step is crucial for making sure that your work is stored and available for future use, whether for sharing, printing, or further editing.
Post-processing and saving the result are the final touches in any image manipulation project. Properly converting and saving the image ensures that your final product looks as intended and is ready for use. This step might seem simple, but it’s essential for maintaining the quality and accuracy of your work.
Practical Applications and Use Cases
AI face swapping technology has a wide range of applications across different fields. Here’s a look at how this technology is being used in various areas, from entertainment to education.
Entertainment and Media
In the world of entertainment and media, AI face swapping is making waves by transforming how content is created and consumed. Here’s how:
Creating Engaging Content:
- Movies and TV Shows: Face swapping can add incredible visual effects to films and TV shows. Imagine placing a classic actor’s face into a modern scene or blending special effects effortlessly. This opens up new creative possibilities for storytelling.
- Video Games: Game developers use face swapping to make characters more customizable. Players can see their own faces in the game or even use famous faces to create a unique gaming experience. This adds a personal touch and deeper immersion than typical character designs.
- Social Media: Influencers and content creators use face swapping to make entertaining, shareable content. From hilarious memes to interactive posts, this technology helps grab attention and boosts engagement on social platforms.
Marketing and Advertising:
- Personalized Campaigns: In advertising, face swapping can be used to create personalized ads. By inserting a consumer’s face into an ad, it becomes more relatable and engaging, increasing the chance of conversion.
- Humorous Content: Marketers often use face swapping to add humor to their campaigns. Swapping faces in funny or surprising ways can capture attention and make an ad memorable. This playful twist can help improve brand recognition.
Educational and Research Applications:
- Demonstrating AI Capabilities: In educational settings, face swapping is a great way to showcase how AI technologies work. It demonstrates the power of machine learning and computer vision in a way that’s easy to understand, making complex topics more accessible.
- Research and Development: Researchers use face swapping technology to test and improve algorithms and models. It provides a controlled environment for studying how different methods perform, helping to advance AI and enhance the accuracy of applications.
Ethical Considerations and Best Practices
Ethical Use of Face Swap Technology
Respecting Privacy and Getting Permission:
- Respect Privacy: Before using someone’s face in a face swap, always ask for permission. This applies whether the person is a public figure or a private individual. Protecting their privacy is important for trust and avoiding legal problems.
- Be Clear About Usage: Let people know exactly how their face will be used in the project. Being upfront about where and how their image will appear ensures they understand the situation and prevents misuse.
Avoiding Misuse of the Technology:
- Prevent Harmful Use: Face swapping can be used to create misleading or harmful content. It’s important to have measures in place to avoid using the technology for creating deepfakes or spreading false information that could hurt people’s reputations.
- Create Ethically: Use face swapping responsibly. Avoid creating content that might be offensive or misleading. Using the technology in a respectful and ethical way helps maintain its credibility and trustworthiness.
Best Practices for Realistic Face Swaps
Achieving High-Quality and Realistic Results:
- Pay Attention to Detail: To create a realistic face swap, make sure all the details are accurate. This includes matching colors, facial expressions, and overall blending. A great face swap looks natural and believable.
- Use Advanced Tools: To get the best results, use the latest face swapping techniques and tools. These can include deep learning models and new software that enhance the final result’s realism.
Staying Up to Date:
- Keep Learning and Updating: AI and face swapping technology are constantly improving. Stay updated with new tools and methods to ensure your work is using the best and most effective techniques.
- Invest in Learning: Take the time to learn about new developments in face swapping. Keeping your skills sharp and understanding the latest ethical guidelines will help you produce high-quality, responsible work.
Conclusion
In this guide, we’ve covered the main aspects of AI face swapping. We started by explaining what AI face swapping is and how it’s different from regular image editing. Then, we explored the technology behind it, including deep learning algorithms, Generative Adversarial Networks (GANs), and facial recognition. We also talked about the importance of face alignment and preparation, ensuring that images are ready for the best results.
We looked at different tools and libraries that help with face swapping, like DeepFaceLab, FaceSwap, and open-source libraries such as OpenCV, dlib, and frameworks like TensorFlow and PyTorch.ed a practical step-by-step guide for implementing face swaps, including loading and preparing images, face detection and alignment, and post-processing.
Future Trends in AI Face Swaps
AI face swapping is improving quickly, and there are some exciting things coming soon:
Better Realism: We can expect face swaps to look even more real in the future. Thanks to improvements in AI and image technology, the results will get more lifelike and accurate.
Ethical Improvements: As the technology gets better, there will also be more focus on using it responsibly. We will likely see better rules and tools to make sure it’s used in the right way, reducing risks and misuse.
AR and VR Integration: Combining face swapping with Augmented Reality (AR) and Virtual Reality (VR) will open up new, immersive experiences, like virtual try-ons and interactive stories.
Easier Access: Future improvements in tools and platforms will make face swapping easier for more people. This will allow a wider audience to explore and create with this technology.
Take Action
Now that you’ve learned about AI face swapping, it’s time to try it out for yourself. Start experimenting with the tools and techniques we’ve covered, and see what creative ideas you can come up with. Whether you’re looking to enhance content, create personalized ads, or just explore AI, there are many exciting opportunities ahead.
Start your own projects, share your creations, and connect with others who are interested in AI and face swapping. The journey is just beginning, and the possibilities are endless. Explore, create, and have fun bringing your ideas to life with AI!
External Resources for Creating Realistic Face Swaps with AI
Tools and Libraries
- DeepFaceLab
- An open-source tool for creating realistic face swaps, with detailed documentation and community support.
- Visit DeepFaceLab
- FaceSwap
- An open-source project for face swapping, featuring comprehensive guides and a supportive community.
- Visit FaceSwap
- OpenCV
- A library for computer vision tasks, including face detection and manipulation.
- Visit OpenCV
- dlib
- Provides tools for facial landmark detection and alignment, crucial for accurate face swapping.
- Visit dlib
- TensorFlow
- A framework for building and training AI models, useful for custom face swapping applications.
- Visit TensorFlow
- PyTorch
- An alternative to TensorFlow, with tools for developing deep learning models for face swapping.
- Visit PyTorch
FAQs
AI face swapping is a process where artificial intelligence algorithms replace one person’s face with another’s in an image or video. This technique uses advanced machine learning models, such as Generative Adversarial Networks (GANs), to create realistic and convincing face swaps.
Face swapping technology involves several steps:
Face Detection: Identifying faces in images or videos using algorithms.
Face Alignment: Aligning and standardizing the detected faces for consistent swapping.
Face Replacement: Using AI models, like GANs, to blend the target face onto the source face seamlessly.
Post-Processing: Refining the swapped face to match the original image’s lighting, color, and texture.
Key tools and libraries for face swapping include:
DeepFaceLab: A popular tool for creating high-quality face swaps.
FaceSwap: An open-source project for face swapping with detailed documentation.
OpenCV: Used for image processing tasks like face detection.
dlib: Provides facial landmark detection and alignment.
TensorFlow and PyTorch: Used for building and training custom AI models.
To set up your environment for face swapping, you need to:
Install Python and package managers like pip or conda.
Install Required Libraries: Such as TensorFlow, OpenCV, and dlib.
Prepare Your Dataset: Collect and organize images for training and swapping.
Configure Tools: Follow specific setup instructions for tools like DeepFaceLab or FaceSwap.
Ethical considerations include:
Privacy and Consent: Always obtain explicit permission from individuals whose faces are being used.
Avoiding Misuse: Prevent the creation of misleading or harmful content.
Transparency: Clearly communicate how and where the swapped faces will be used.
Leave a Reply